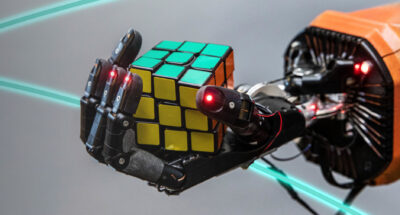
How will Generative AI impact jobs and productivity?
Mike and Amit talk about the impact generative AI will have on the global economy and how long it will take for change to come....
by Heather Cairns-Lee, Sarah E. Toms Published 1 December 2023 in Technology • 9 min read
In business, effective communication is the bedrock of success, as is widely acknowledged in various studies. According to the 2023 State of Business Communication report, business leaders attribute heightened productivity (72%), increased customer satisfaction (63%), and boosted employee confidence (60%) as the top three advantages resulting from effective communication. Â
However, the study also reveals a decline in communication effectiveness (down 12%) and a resultant 15% drop in productivity. The repercussions are substantial: poor communication is estimated to incur an annual cost of $1.2 trillion for businesses in the US, averaging $12,506 per employee per year. It’s an issue we hear consistently from executives at IMD in Switzerland, and one they’re keen to address.
While challenges persist in human-to-human communication, introducing powerful machines, such as new forms of artificial intelligence (AI), adds another layer to the conversation. How are we communicating with these machines, and are there ways to communicate more effectively?
Undoubtedly, AI has the potential to revolutionize business communication. New forms not only enhance productivity, freeing up time for the creative elements of communication, they also generate content and improve accessibility for individuals with disabilities through speech-to-text technologies.
However, responsible use of AI in communication requires ethical considerations, transparency, and a balance between technological efficiency and human-centric approaches. Still, successful human-AI collaboration is already well underway. Early studies suggest considerable improvements in productivity through written communication for those who embrace the latest wave of ground-breaking generative AI tools, such as OpenAI’s GPT-4.
The first step is understanding this much-hyped technology. Generative AI (GenAI), a sub-field of machine learning, is a marvel that may take us a decade to fully comprehend. It operates by generating new content and data based on a human prompt, which can originate from humans through text or voice prompts, or from other machines through code.
Large Language Models (LLMs) power the resulting output, whether in the form of text, images, code, videos, or music. This output often feels like our own, as the creation process involves our questions and inquiries to the AI. However, the quality of the output is largely driven by the quality of the questions, also known as prompts, that we provide.
One challenge in human-AI interaction is bias in the data. Four biases are important for business executives to be aware of.
Completion bias occurs when AI responses appear so authoritative and articulate that users accept them unquestioningly, failing to scrutinize them for potential shortcomings. The risk here is that organizations may experience a decline in innovative thinking if business ideas are merely a regurgitated response from an AI chatbot without further analysis and creative thinking.
Confirmation bias materializes when an AI system confirms pre-existing beliefs or biases held by users, effectively providing support for favored interpretations. An example of this is when an AI system predicts a job candidate’s success based on biases held by the hiring manager.
Anchoring bias is the tendency to adjust judgments toward the first piece of information that is experienced. The danger with this bias is that unless business executives rigorously examine the data from AI, they may attach to the first information returned, especially if this confirms their pre-existing views.
Sampling bias happens when the data is not representative of the population it serves, leading to bias for certain groups. For example, facial recognition trained mostly on white people may not recognize people of other races.
To avoid these biases in our interactions with GenAI, it is imperative to strike a balance between accepting information from chatbots and probing further for possible limitations. Business leaders play a pivotal role in this process by analyzing and interrogating information before applying it. This approach involves learning to ask the right questions and using multiple queries to encourage the AI to generate higher-quality answers.
Posing better questions enhances communication and fosters critical and creative thinking, deemed crucial skills by the World Economic Forum’s Future of Jobs 2023 report. Critical thinking involves asking effective questions, challenging assumptions, researching, and analyzing evidence. Creative thinking is nurtured by curiosity, multiple perspectives, and the habit of questioning. Both skills are essential to effective management and communication, and both rely on the ability of business leaders to ask effective questions.
So how do we apply critical and creative thinking to the co-generation process with GenAI?
It is imperative to preserve your voice when using this technology in your business communications. People will question whether ideas and communication originate from colleagues, or whether they are simply cut-and-paste responses from GenAI. So, ensure that your communication remains authentic by collaborating with AI for productivity gains and then adding your unique voice and thoughts to the output it generates.
The technology is clearly not without its pitfalls, including the potential to stifle creative thinking through over-reliance on AI, the proliferation of hallucination (when GenAI makes up plausible-sounding answers), and the generation of poor-quality data based on inadequate queries. The solution is to focus on asking better questions. Questions are powerful; they stimulate deeper thinking, engage the brain, and ignite the quest for knowledge.
In this quest, clean language – an approach that minimizes assumptions – can be profoundly beneficial. By paying attention to embedded assumptions in our questions, we can fine-tune our queries and interact more effectively with GenAI. Clean Language Interviewing (CLI) is built on the principle of avoiding asking “leading” questions, resulting in potentially less bias in data. Unlike open-ended questions, leading questions often carry implicit assumptions or suggest a preferred answer.
This may sound easy yet research shows that identifying and avoiding leading questions is an underestimated challenge, requiring deliberate attention and practice. This is particularly important for business leaders who ask a disproportionate number of questions to support decision-making. Unaware of how their questions might lead or introduce bias, they risk fostering echo chambers that perpetuate the biases discussed earlier in this article.
CLI serves to reduce biases from inquiries, rendering it a valuable tool applicable across various domains, such as business, coaching, conflict resolution, and market research. For business leaders, the advantages of CLI include fostering self-awareness, minimizing bias, and enhancing communication.
Another valuable tool for understanding knowledge creation is “Bloom’s taxonomy” and its 2001 revised edition, which presents a hierarchical model of cognitive skills across six levels: remembering, understanding, applying, analyzing, synthesizing, and evaluating. Applying this model to business questions for GenAI fosters more sophisticated responses. Progressing from Level I to Level VI involves increasing complexity and demands higher-order thinking, enhancing leaders’ focus on details and collaboration with AI.
This is the basic level of learning where you acquire information. In a business context, this could involve learning about your industry, your competitors, or new technologies. Â
At this level, you comprehend the information you’ve learned. For a business leader, this could mean understanding how a new regulation affects your business. Â
This involves using what you’ve learned in new situations. For example, you might apply a new marketing strategy that you’ve learned about or use a new project management tool. Â
Here, you break down complex information into parts for better understanding. This could involve analyzing market trends or financial reports, and then drawing inferences and seeking substantiating evidence. Â
This involves combining information to form a new whole. In business, you might develop a new business strategy based on market analysis, or create a new product based on customer needs. Â
At this level, you make judgments about the value of ideas. This could involve evaluating the effectiveness of a marketing campaign, the performance of a product, or the feasibility of a new business strategy. Â
Ultimately, as GenAI becomes an integral part of our professional lives, the skill of asking questions takes on greater significance. Effective questioning can unlock the potential of AI, mitigate biases, and enhance communication, creativity, and problem-solving. It is through the art of asking questions that business leaders can drive effective human-machine interactions and co-creation.Â
Affiliate Professor of Leadership and Communication
Heather Cairns-Lee is Affiliate Professor of Leadership and Communication at IMD. She is a member of IMD’s Equity, Inclusion and Diversity Council and an experienced executive coach. She works to develop reflective and responsible leaders and caring inclusive cultures in organizations and society.
Chief Learning Innovation Officer
Sarah Toms is Chief Learning Innovation Officer at IMD where she leads the Learning Innovation and AI strategy. Sarah previously co-founded Wharton Interactive, an initiative at the Wharton School that has scaled globally. A demonstrated thought leader in the educational technology field, she is fueled by a passion to find and develop innovative ways to make every learning environment active, engaging, more meaningful, and learner-centric. Sarah is an AWS Education Champion, and has been on the Executive Committee of Reimagine Education for 8 years. She has spent more than 25 years working at the bleeding edge of technology, and was an entrepreneur for over a decade, founding companies that built global CRM, product development, productivity management, and financial systems. In addition, Sarah is coauthor of The Customer Centricity Playbook, the Digital Book Awards 2019 Best Business Book.
15 November 2023 • by Amit M. Joshi, Michael R. Wade in Artificial intelligence
Mike and Amit talk about the impact generative AI will have on the global economy and how long it will take for change to come....
2 November 2023 • by Goutam Challagalla in Artificial intelligence
Generative AI can transform marketing, customer service, and other business areas, but CEOs must ensure they use it effectively, says IMD’s Goutam Challagalla ...
26 October 2023 • by Faisal Hoque in Artificial intelligence
Ground-breaking developments in immunotherapy benefit greatly from the introduction of AI while diagnosis is more precise and cost-effective. ...
19 October 2023 • by Michael R. Wade, Amit M. Joshi in Artificial intelligence
Mike and Amit discuss which of the tech giants will one day dominate generative AI...
Explore first person business intelligence from top minds curated for a global executive audience